Leveraging AI for Scientific Knowledge Extraction
AI is transforming the analysis of extensive biomedical data, allowing pharma companies to expedite R&D processes, and cut costs. By reaching conclusions quicker, the inclusion of AI in drug development pipelines can inform decision-making, enabling the prioritization of more promising research avenues.
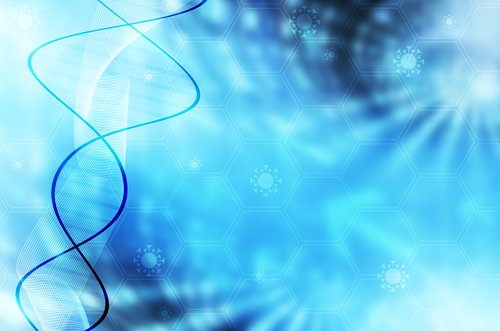
The Cost of Drug to Market is Increasing
In today’s pharmaceutical landscape, bringing a single drug to market costs an average of $1.4 billion out-of-pocket costs and a decade of research, reflecting an upward trajectory in development costs.¹ The sheer volume of scientific data, complexities of diseases, and stringent regulatory requirements further complicate drug development. Researchers must sift through extensive data to identify targets and drug candidates, spending around 23% of total work time reading.² Moreover, leading research-based pharma companies have had to compensate for negative R&D productivity through mergers and acquisitions.³ But what if you could accelerate the critical path from disease biology to candidate selection? This is where AI can help.
The Impact of AI in Drug Discovery
In drug development, the challenge lies in the selection of candidates with the highest success potential, demanding rigorous analysis. AI emerges as a pivotal technology, with applications spanning the drug development lifecycle, from discovery to postmarket safety surveillance and manufacturing.⁴ Key applications of AI in drug discovery encompass toxicity prediction, target identification, drug repurposing, and de novo drug design.⁵ Leveraging AI for scientific knowledge extraction has the potential to improve the assessment of drug targets, as well as better prioritize viable indications to target.¹ In this context, we illustrate how Causaly’s capabilities are instrumental in realizing these improvements, bridging the gap between AI and millions of scientists worldwide.
Identifying Targets from the Biomedical Literature
Causaly can aid investigations into the identification of promising targets to pursue in drug development. As an example, we used Causaly to explore targets for chronic pancreatis, a progressive inflammatory disease with no cure.
By machine-reading the entire volume of biomedical literature, more than 1,400 targets associated with chronic pancreatitis were uncovered. Results can be visualized as a dendrogram of results, allowing users to peruse evidence for the target-disease relationship.
To focus on targets with genetic evidence, results were filtered by the GWAS catalog data source, unveiling 33 potential targets for chronic pancreatitis. Examples of targets with compelling evidence within this criterion include TRPV, SPINK1, and RNF128.⁶
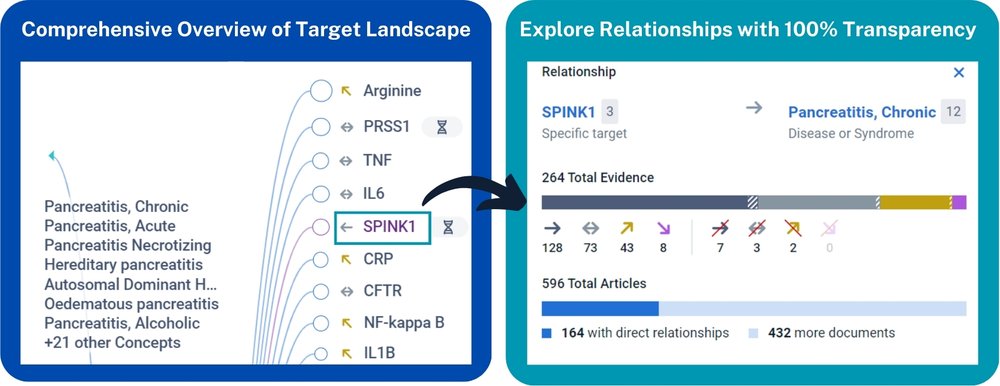
Potential Mediators of the Target-Disease Effect
Once a target of interest has been identified, users can deep-dive into the pathways that may mediate the effect of a target on chronic pancreatitis using the multi-hop module. As an example, we used the hypothesis generation tool to identify 21 proteins which may mediate the effect of SPINK1 on chronic pancreatitis (Figure 2). Similarly, 11 pathways which may connect SPINK1 and chronic pancreatitis were identified. Such insights can provide the foundation for a hypothesis.

Fail Early, Fail Fast
AI is transforming the analysis of extensive biomedical data, allowing pharma companies to expedite R&D processes, and cut costs. By reaching conclusions quicker, the inclusion of AI in drug development pipelines can inform decision-making, enabling the prioritization of more promising research avenues. This strategy is an efficiently tackles the literature overload, embodying the “Fail Early, Fail Fast” approach to preclinical research, thereby saving time and resources in drug development.
References
- McKinsey & Company, Generative AI in the pharmaceutical industry: Moving from hype to reality, 2024. Source
- Hubbard, K. E., Dunbar, S. D., PLoS One., 2017;12(12):e0189753. Source
- Schuhmacher, A., Hinder, M., et. al., Drug Discov. Today., 2023;28(10):103726. Source
- FDA, Artificial Intelligence and Machine Learning (AI/ML) for Drug Development, 2023. Source
- Vora, L. K., Gholap, A. D., Jetha, K., et. al., Pharmaceutics, 2023;15(7):1916. Source
- Rosendahl, J., Kirsten, H., Hegyi, E., et. al., Gut., 2018;67(10):1855-1863. Source
Further reading
Get to know Causaly
What would you ask the team behind life sciences’ most advanced AI? Request a demo and get to know Causaly.
Request a demo