Biomarkers of Treatment Response in Liver Cancer
Biomarkers serve as objective measures of treatment response to guide patients towards the most appropriate therapies. Yet, in the era of big data, pinpointing promising biomarkers remains a challenging endeavor. AI is revolutionizing translational medicine by improving the efficiency and accuracy of biomarker identification.
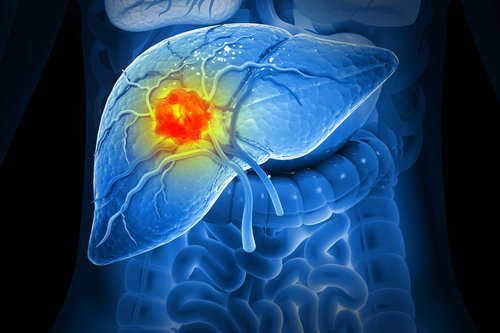
AI: Revolutionizing Translational Medicine
Biomarkers serve as objective measures of treatment response to guide patients towards the most appropriate therapies and minimizing unnecessary treatments. Yet, in the era of big data, pinpointing promising biomarkers remains a challenging endeavor. AI is revolutionizing translational medicine by improving the efficiency and accuracy of biomarker identification. Here, we used Causaly’s human-centric AI to identify and prioritize sorafenib and lenvatinib treatment response biomarkers in hepatocellular carcinoma (HCC).
Treating Hepatocellular Carcinoma (HCC)
HCC, often diagnosed at advanced stages with limited curative options,¹ has a concerning 5-year survival rate of just 10% in the U.S.² The choice of treatment is therefore imperative. Treatment modalities for HCC include liver transplantation, resection and radiofrequency ablation,³ and systemic drugs including sorafenib and lenvatinib.⁴ In this example, we utilized Causaly Cloud to explore treatment response biomarkers to sorafenib and lenvatinib in HCC.
Identifying Biomarkers of HCC
Using Causaly Cloud, 9,000+ biomarkers for HCC were extracted from the entire volume of biomedical literature, as visualized in the dendrogram in Figure 1.

Biomarkers of Treatment Response
Around 150 biomarkers of treatment response in HCC were identified: 26 biomarkers were related to sorafenib response, and 4 biomarkers for levatinib response. After perusing the supporting evidence, biomarkers of interested were selected.
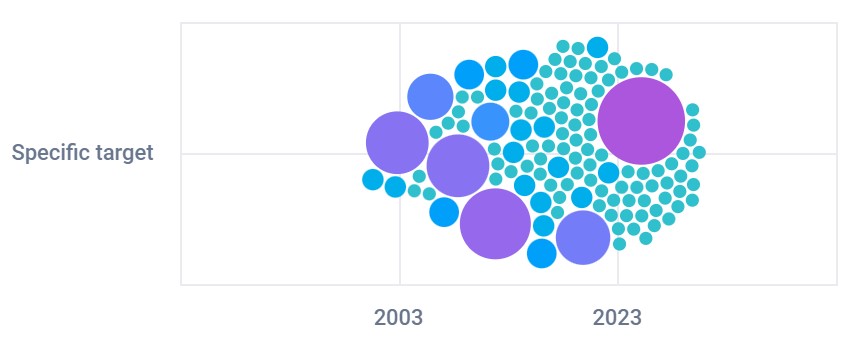
Sorafenib Treatment Response
Two biomarkers of sorafenib treatment responses with the strongest evidence were runt-related transcription factor 2 (RUNX2) and fibroblast growth factor 19 (FGF19).
- RUNX2 - A study demonstrated that gene splicing of RUNX2 hindered cell growth and migration and improved Hep3B cell response to sorafenib treatment, highlighting the potential role of the RUNX2 in HCC chemoresistance.⁵
- FGF19 – A 2017 CRISPR study demonstrated the role of FGF19 in HCC resistance to sorafeib therapy. In this study, the overexpression of FGF19 was shown to inhibit the effect of sorafenib on apoptosis and reactive oxygen species generation.⁶
Lenvatinib Treatment Response
Of the identified levatinib treatment response biomarkers, AFP and angiopoietin-2 (Ang-2) were selected for further exploration.
- AFP – A study investigated the clinical features of unresectable HCC reported favourable early therapeutic response to lenvatinib.⁷ Follow-up imaging showed a significant decline in log10 AFP from the baseline, suggesting AFP may have a predictive value in patients receiving lenvatinib treatment.⁷
- Ang-2 – Reduced levels of Ang-2 were observed in blood samples obtained from lenvatinib-treated HCC patients.⁸ This suggests that early changes in Ang-2 levels could be predictive biomarkers of clinical response to levatinib therapy in HCC.⁸
Conclusion
The exploration of biomarkers of treatment response is pivotal to advancing personalized medicine. Such biomarkers offer promising avenues for predicting therapeutic efficacy and tailoring treatments to enhance outcomes for patients.
More on cancer biomarkers here
References
- Sim, H. W., Knox, J., Curr. Probl. Cancer., 2018;42(1):40-48. Source
- Golabi, P., Fazel, S., Otgonsuren, M., et. al., Medicine (Baltimore)., 2017;96(9):e5904. Source
- Raza, A., Sood, G. K., World J. Gastroenterol., 2014;20(15):4115-27. Source
- Luo, J., Gao, B., Lin, Z., et. al., Front. Oncol., 2022;12:1010726. Source
- Emma, M. R., Iovanna, J. L., Bachvarov, D., et. al., Cell Death Dis., 2016;7(6):e2269. Source
- Gao, L., Wang, X., Tang, Y. et al., J. Exp. Clin. Cancer Res., 2017;36(1):8. Source
- Hiraoka, A., Kumada, T., Kariyama, K., et. al., Cancer Med., 2019;8(1):137-146. Source
- Chuma, M., Uojima, H., Numata, K., et. al., Cancers (Basel)., 2020;12(2):293. Source
Further reading
Get to know Causaly
What would you ask the team behind life sciences’ most advanced AI? Request a demo and get to know Causaly.
Request a demo