Eliminating Bias with Human-Centric AI
Traditional keyword searching can miss crucial information, skewing representations of research landscapes before reading even begins. Instead, Causaly’s human-centric AI can extract all targets for a given disease without bias, uncovering scientific insights, rather than just papers.

The Problem with Traditional Keyword Searching
Traditional keyword searching involves entering specific words or phrases into a search engine, typically returning hundreds or thousands of papers with these keywords in their titles or abstracts.¹ However, keyword ambiguity and lack of context can yield irrelevant results,² making it difficult the separate the signal from the noise. Faced with this, most scientists resort to reading just the title and abstracts, introducing selection bias into the papers they choose to read. Additionally, keyword searching can miss crucial information, leading to skewed representation of research landscapes.
Causaly: A Differentiated AI Solution
Causaly’s AI solution reads the entire volume of biomedical literature and data in seconds, finding answers to complex research questions without bias. By machine-reading the literature, Causaly separates the signal from the noise, extracting only the most relevant insights from comprehensive data sources. Importantly, Causaly is fully transparent, providing users with all source information, enabling users to apply their own judgement to supporting evidence. As an example, we used Causaly Cloud to identify and prioritize targets for gout, a form of arthritis which causes severe joint pain.
Uncover Entire Target Landscapes
Using Causaly, almost 700 targets for gout were identified, extracted from 1,600+ supporting documents. All targets can be visualized as a dendrogram view of results, showing the type of target-disease relationship, and additional information including GWAS or clinical data.
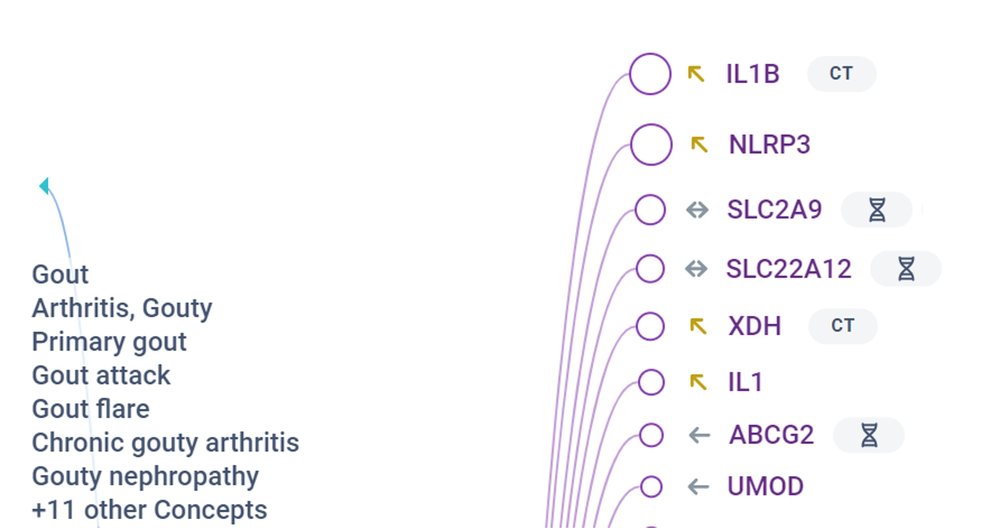
Prioritize Targets by Desired Criteria
Using Causaly’s advanced filtering capabilities, targets can be prioritized by desired criteria including by GWAS studies, clinical trials, primary data, animal models and novelty (Figure 2).

- GWAS: 70+ Targets for gout have been reported in the GWAS catalog. ABCG2 was identified as target with compelling evidence for gout, with a GWAS study implicating rs4148155 as a significant gout loci.³
- Clinical Trials: 10 Targets for gout reported on clinicaltrials.gov. IL1β was identified as a target for gout investigated in clinical trials. A phase Ib/II study of recombinant anti-IL-1β injection in Chinese participants with acute gout is ongoing.⁴
- Primary Data: 400+ targets for gout were extracted from primary data. TRPM2 was among targets with strong evidence, with TRPM2 deficiency shown to significantly impair inflammatory response induced by monosodium urate crystals (the causal agent of gout).⁵
- Animal Studies: Around 33% of targets identified by Causaly have been studied in animal models. The pharmacological inhibition of IRAK4, for example, has shown to block gout formation in mouse models.⁶
- Emerging Targets: ~150 targets for gout have been reported in the literature since 2023 to date. A recent study concluded that rare, damaging variants of LDHD can cause autosomal recessive early-onset gout.⁷
Human-Centric AI vs. Keyword Searching
Searching “Targets for gout” in PubMed returned more than 1,500 results. Instead, Causaly’s human-centric AI uncovers the entire target landscape (~700 targets from 1,600+ documents), extracting relevant scientific insights, rather than just papers, from multiple data sources. By eliminating bias, researchers can make better-informed decisions, thereby enhancing the success rate of new drug development programs.
References
- Ma., J., Wu, Z., Huang, L., Sci. Program., 2022; 2022(1). Source
- Karimi, K., Agalakov, S., Telmer, C. A., et. al., Database, 2021;2021(1). Source
- Nakayama, A., Nakatochi, M., Kawamura, Y., et. al., Ann. Rheum. Dis., 2020;79(5):657-665. Source
- ClinicalTrials.gov. NCT05588908. Retrieved 9th January 2024. Source
- Zhong, Z., Zhai, Y., Liang, S., et. al., Nat. Commun., 2013;4(1):1611. Source
- Kelly, P. N., Romero, D. L., Yang, Y., et. al., J. Exp. Med., 2015;212(13):2189-201. Source
- Bardin, T., Ducrot, Y. M., Nguyen, Q., et. al., Rheumatol. (Oxford), 2023;62(12):3978-3983. Source
Further reading
Get to know Causaly
What would you ask the team behind life sciences’ most advanced AI? Request a demo and get to know Causaly.
Request a demo