Target Identification and Prioritization: Ovarian Cancer
Human-centric AI can be used to accelerate target identification and prioritization, extracting evidence for the most relevant target-disease relationships. Importantly, our AI provides a view of all scientific evidence, ensuring 100% traceability or original sources for researchers to apply their own judgement.
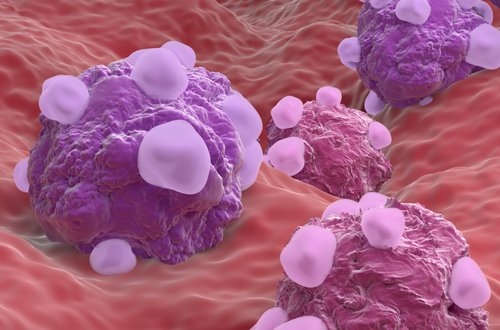
High-Grade Serous Ovarian Cancer
High-grade serous ovarian cancer (HGSC) is the most aggressive subtype of serous ovarian carcinoma. Exhibiting little to no clinical presentation until the disease has progressed,¹ HGSC has a 10-year survival rate of just 15% at advanced stages.²
The Need for Targeted Therapies
At diagnosis, HGSCs exhibit favourable response to platinum-based drugs, but poor selectivity and growing chemotherapeutic resistance is a concern, highlighting the need for targeted therapies to improve patient outcomes. Pivotal to this process is the identification of genetic mutations or resultant abnormal proteins.³
Human-centric AI can be used to accelerate target identification and prioritization, extracting evidence for the most relevant target-disease relationships. Importantly, our AI provides a view of all scientific evidence, ensuring 100% traceability of original sources for users to apply their own judgement. Here, we demonstrate how Causaly can be used to explore potential therapeutic targets for HGSC.
Target Identification and Prioritization
By machine-reading the entire volume of biomedical literature, Causaly can rapidly identify targets for a given disease. Here, 6,500+ targets for ovarian cancer were identified, over 700 of which were reported for the HGSC subtype.
Using Causaly’s advanced filtering capabilities, targets can be refined by different criteria, including by study type, primary data, publication type, journal impact factor, target-disease relationship, and novelty (Figure 1).

Explore Target Insights
- Animal Models: A study using a mouse model of HGSC demonstrated the positive impact of STAT1 induced T cell recruiting on the tumor immune microenvironment of HGSC.⁴
- Primary Data: In a preclinical study, data showed a favourable impact of high XIAP expression on the outcome of platinum-treated HGSC patients.⁵
- Clinical Trial Publications: A recent study showed that elevated cytoplasmic and nuclear BMP7 expression was significantly associated with HGSC suggesting a role in aggressive phenotypes.⁶
- Top 25% Journals: In the absence of BRAF mutations, the MAP3K8 protein was identified as a prognostic marker and therapeutic target in HGSC.⁷
- Relationship Type: The expression of short WDFY2 protein may alter the PI3K and AKT pathway, which may in turn upregulate tumor progression in HGSC.⁸
- Emerging: A 2023 study demonstrated that VGLL3 protein expression a nuclear and cytoplasmic level were significantly higher in HGSC tissues compared to normal tissues.⁹
Conclusion
The aggressive nature and late-stage clinical presentation of HGSC demands the novel, targeted therapeutics to improve disease management and patient outcomes. The discovery of relevant therapeutic targets for HGSC is the first step in this process. Causaly can accelerate this process by rapidly identifying targets from biomedical literature, enabling researchers to uncover hidden connections and explore novel avenues.
References
- Liberto, J. M., Chen, S. Y., Shih, I. M., et. al., Cancers (Basel)., 2022;14(12):2885. Source
- Narod, S., Nat. Rev. Clin. Oncol., 2016;13(1):255–261. Source
- Shuel, S. L., Can. Fam. Physician., 2022;68(7):515-518. Source
- Au, K. K., Peterson, N., Truesdell, P., et. al., Gynecol. Oncol., 2017;145(3):436-445. Source
- Cremona, M., Vandenberg, C. J., Farrelly, A. M., et. al., Br. J. Cancer., 2022;127(3):488-499. Source
- Vasan, R., Yadav, J., Aiyappa-Maudsley, R., et. al., J. Cell. Mol. Med., 2023;27(1): 3378-3387. Source
- Gruosso, T., Garnier, C., Abelanet, S., et. al., Nat. Commun., 2015;6:8583. Source
- Kannan, K., Coarfa, C., Rajapakshe, K., et. al., PLoS Genet., 2014;10(3):1004216. Source
- Haque, R., Lee, J., Chung, J. Y., et. al., Front. Oncol., 2023;13:1152991. Source
Further reading
Get to know Causaly
What would you ask the team behind life sciences’ most advanced AI? Request a demo and get to know Causaly.
Request a demo